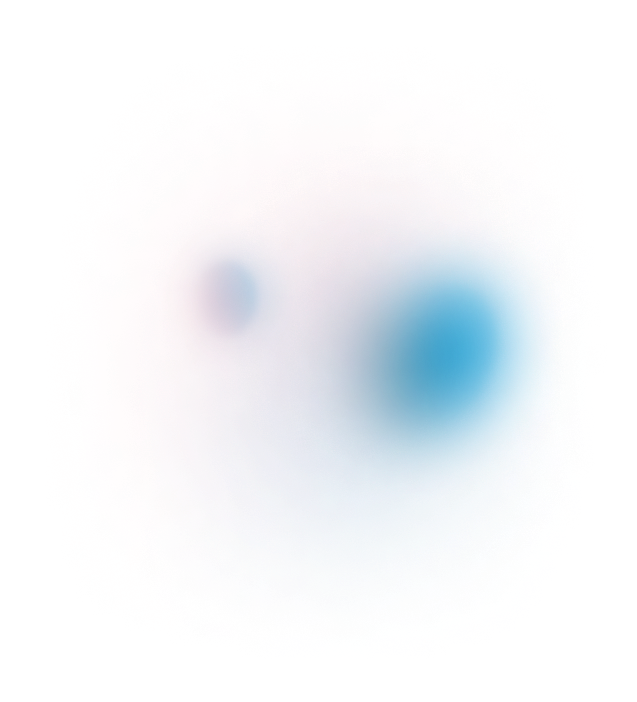
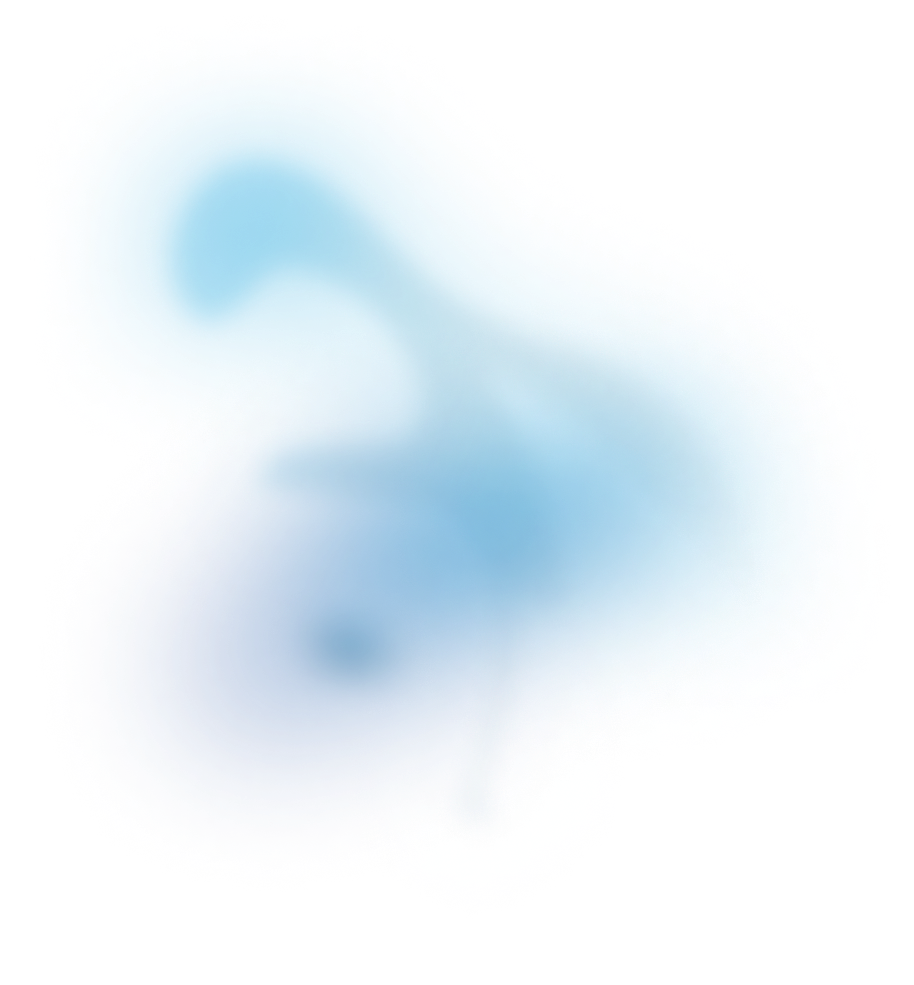
Spatially-Aware Clustering of Ion Images in Mass Spectrometry Imaging Data Using Deep Learning
In this work, we use a pre-trained neural network to extract high-level features from ion images in MSI data, and test whether this improves downstream data analysis
Access publication
Computational analysis is crucial to capitalize on the wealth of spatio-molecular information generated by mass spectrometry imaging (MSI) experiments. Currently, the spatial information available in MSI data is often under-utilized, due to the challenges of in-depth spatial pattern extraction.
In this work, we use a pre-trained neural network to extract high-level features from ion images in MSI data, and test whether this improves downstream data analysis. In this specific work, the resulting neural network interpretation of ion images, which we call neural ion images, are used to cluster ion images based on spatial expressions.
A figure from this work was used as the journal cover image for the special issue on Mass Spectrometry Imaging 2.0 of Analytical and Bioanalytical Chemistry.
We evaluate the impact of neural ion images on two distinct ion image clustering pipelines. In both pipelines, we compare regular and neural ion images from two different MSI datasets. All tested pipelines could extract underlying spatial patterns, but the neural network-based pipelines provided better assignment of ion images, with more fine-grained clusters, and greater consistency in the spatial structures assigned to individual clusters.
The figure below illustrates the biological relevance of the obtained clusters, by relating the mean ion image for three clusters with morphology in a consecutive microscopy section.

Additionally, we introduce the Relative Isotope Ratio metric to quantitatively evaluate clustering quality. Conceptually, the relative isotope ratio measures how well an ion image clustering groups ion images of isotopic mass-to-charge ratios together, thus using orthogonal (spectral) information to assess the clustering quality. The resulting scores show that isotopical m/z values are more often clustered together in the neural network-based pipeline, indicating improved clustering outcomes.
The usefulness of neural ion images extends beyond clustering towards a generic framework to incorporate spatial information into any MSI-focused machine learning pipeline, both supervised and unsupervised.
Publication details
Wanqiu Zhang1,2, Marc Claesen1,2, Thomas Moerman2, Reid M. Groseclose3, Etienne Waelkens4, Bart De Moor1, Nico Verbeeck2,1. Spatially-Aware Clustering of Ion Images in Mass Spectrometry Imaging Data Using Deep Learning, Analytical and Bioanalytical Chemistry 413:, 2803–2819, 2021 - VIEW JOURNAL PAGE
AFFILIATIONS:
- STADIUS CENTER FOR DYNAMICAL SYSTEMS, SIGNAL PROCESSING, AND DATA ANALYTICS, DEPARTMENT OF ELECTRICAL ENGINEERING (ESAT), KU LEUVEN, LEUVEN, BELGIUM
- ASPECT ANALYTICS NV, GENK, BELGIUM
- BIOIMAGING, GLAXOSMITHKLINE, COLLEGEVILLE, UNITED STATES
- DEPARTMENT OF CELLULAR AND MOLECULAR MEDICINE, KU LEUVEN, 3000 LEUVEN, BELGIUM