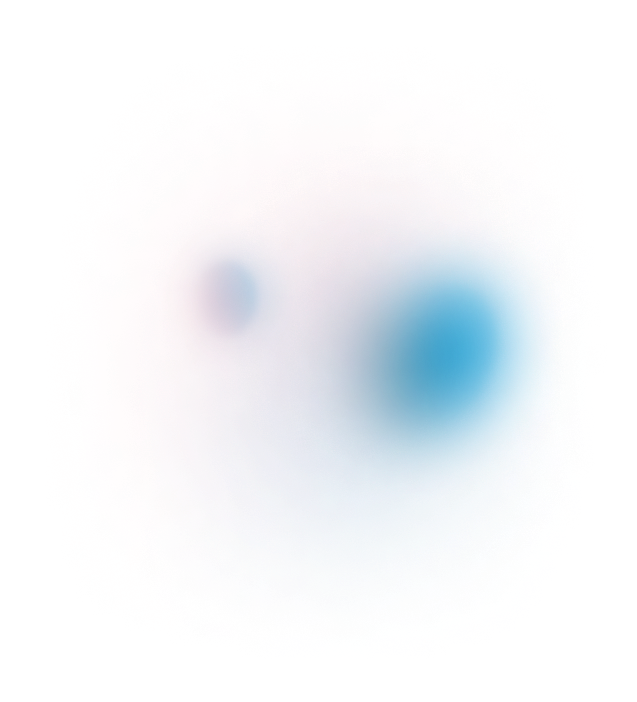
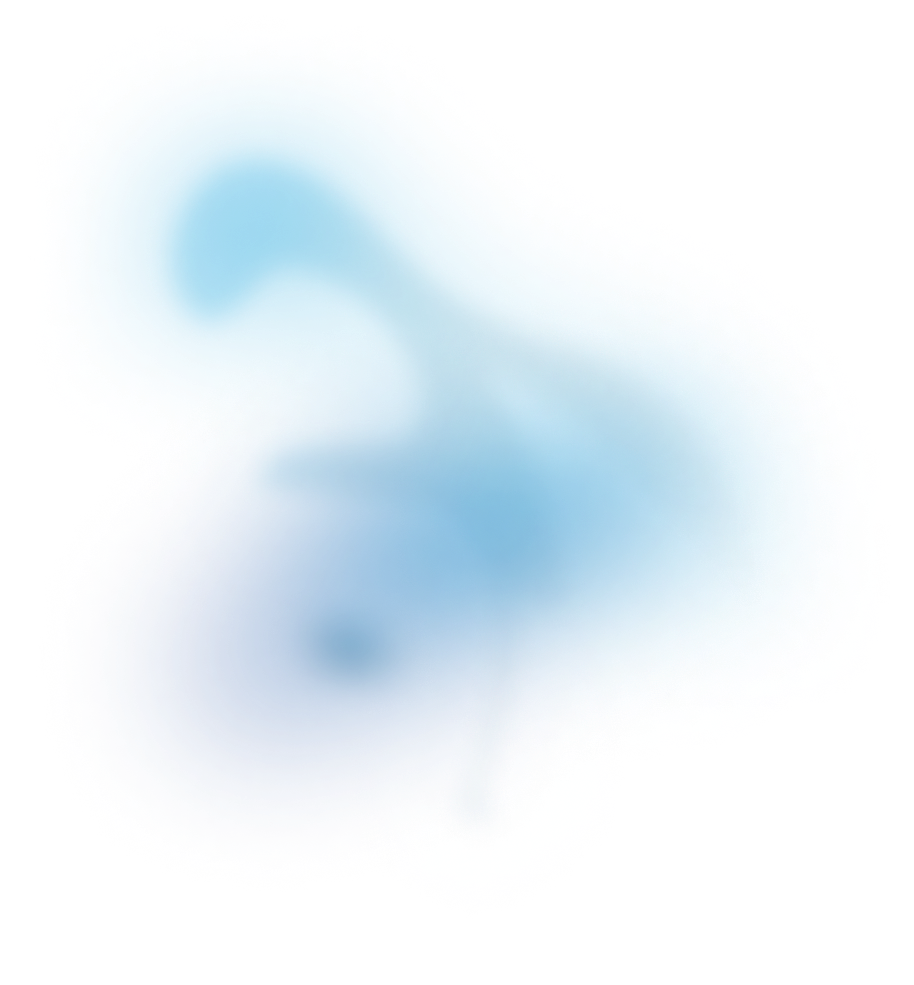
What is spatial multi-omics
It's possible to spatially map proteins, transcripts, lipids and more in tissue samples using spatial omics techniques. When their powers combine, you get spatial multi-omics!
Access publication
Introduction
Just to recap, previous blogs on this website
- has explained some of the basics behind the grand old technique of histology
- explored how some modern tech is becoming spatial, providing additional context e.g. for readouts of transcripts and proteins
- gave a bit of in-depth explanation on how the OG spatial proteomics technique of immunohistochemistry works.
Now we’re going to address the obvious next step – integrating everything together to get spatial multi-omics.
What is multi-omics and why you should do it
Long story short, multi-omics are studies that use different omics analyses to investigate different biological processes relative to Francis Crick’s central dogma of molecular biology.1
While genomics is the study of DNA and genetic information, pretty much all cells in the body have the same genetic content – what makes them different is how the genes are expressed as proteins within given cells, tissues and organs. From here of course we have proteomics and transcriptomics, respectively the study of protein and RNA expression. You might ask – “Why study RNA when you’ve literally just told me proteins are what matter?”, to that I say, “The lack of protein-wide screening method compared to sequencing, and or the lack of suitable antibodies is why transcriptomics might be a good way to answer your question.” Of course, it is also known that transcript content and protein levels don’t necessarily correlate *cough HIF-1 cough*, so combining mRNA and protein measurements are your best bet.
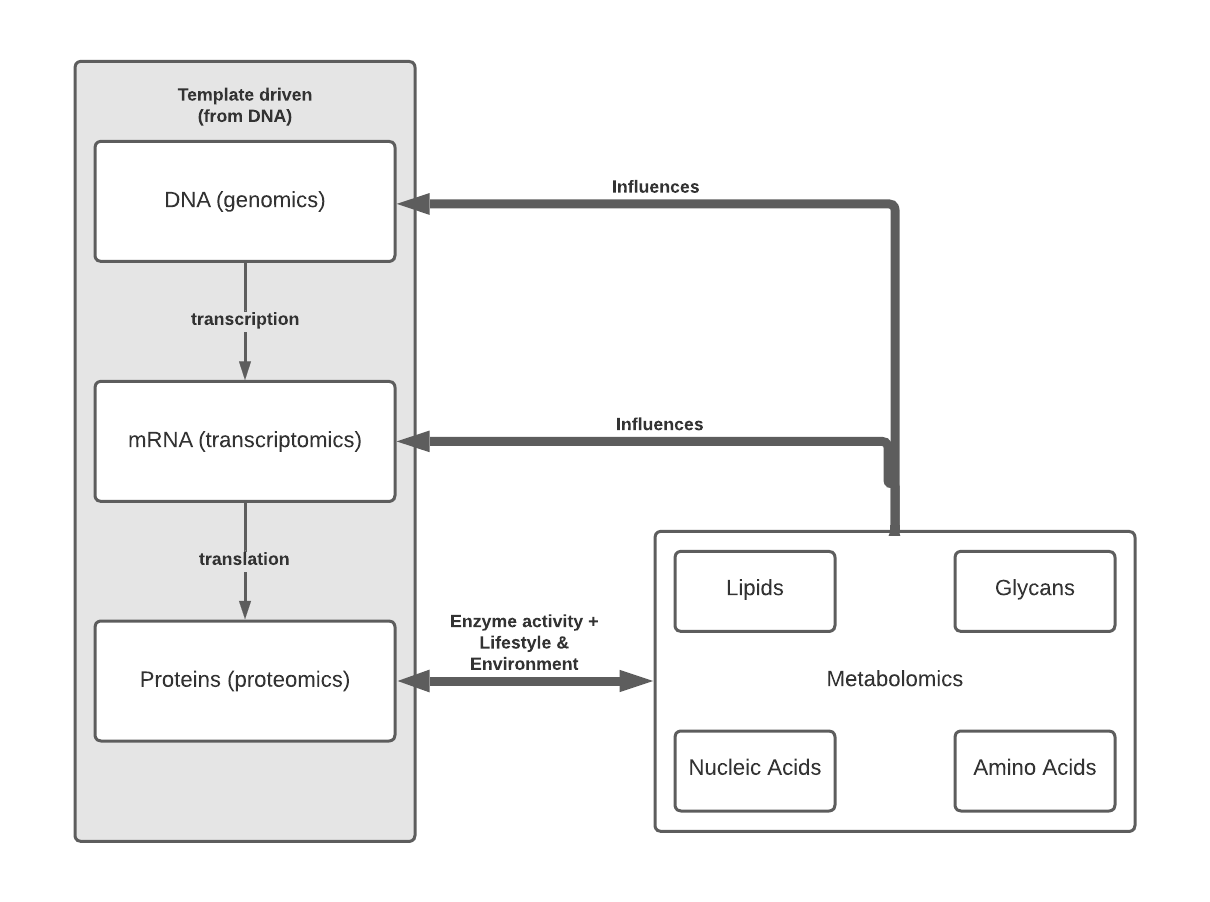
You’ll see that Figure 1 extends beyond the classical idea of central dogma to include metabolomics and metabolomic subdisciplines. Metabolomics is a powerful approach because concentrations of compounds such as small molecule metabolites, lipids etc. reflect not just underlying biochemical activity but also environmental effects. For example, since glycans are synthesized via enzyme activity, not a genetic template, the final form is not fully known until the process is completed, but importantly alterations in glycan structure are known to occur in various diseases and can be mapped to areas of healthy, disease, and disease adjacent tissue.
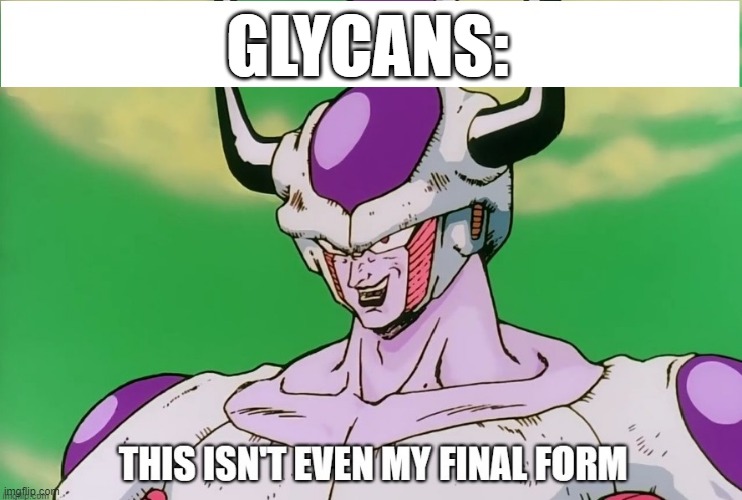
While metabolomics is typically thought of as the final output of the genome and environment, studies are increasingly showing that metabolic pathways and metabolites themselves have a major influence on gene expression. This is through various ways such as:
- Interacting with transcription factors2
- Being the fuel that drives gene expression3
- As a substrate that modulates cell processes e.g. steroid hormones mediating maturation or muscle growth.
While we know there are a wide range of diseases that can arise from or be influenced by environmental factors , a classic example being alcohol-related disease, we’ve also probably heard of someone’s great-aunt Mildred who drank three gin and tonics every day and showed very few ill-effects of that activity. It is rare that a single gene, protein or environmental factor leads to disease development or response to treatment in 100% of the population. Because one omics level will not give a wide enough picture, combining at least two omics analyses is key to interpreting and understanding biological processes - basically what is needed for precision medicine.
Arise, spatial multi-omics!
I’ve said before that context matters so I won’t go over it again. Combining multiple spatialomics analyses is occurring more and more frequently, as seen in both the scientific instrument landscape and in research literature. At this point in time, the most common combination is spatial transcriptomics + proteomics, as seen in techniques that combine RNA sequencing with antibody labelling, such as DBiT-Seq4, CITE-Seq5 and REAP-Seq, but also commercially available instruments - a number of vendors emphasizing that their platforms can be used for detection of proteins and transcripts (e.g. Lunaphore COMET, NanoString CosMx).
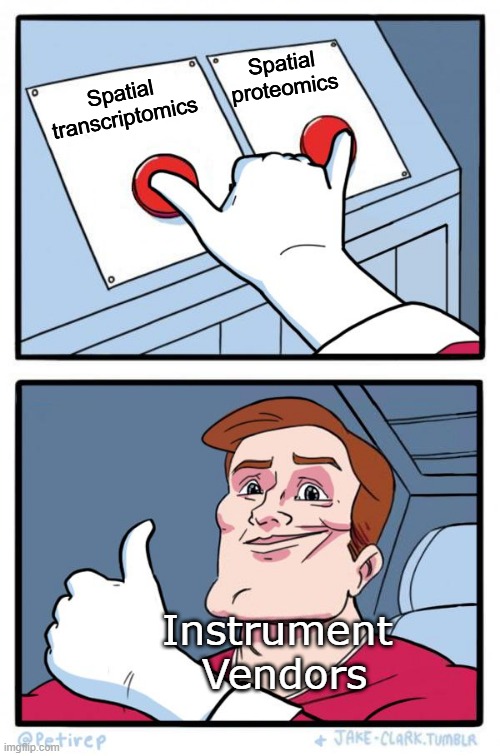
But researchers haven’t stopped at just those two microscopy-based omics techniques! Recent studies have combined spatial transcriptomics and/or proteomics with metabolomic measurements using mass spectrometry. One group combined multi-spectral multiplexed immunofluorescence with MALDI imaging of lipids to investigate cell heterogeneity and immediate molecular context in tissue7. Applied to sections of mouse spleen and atherosclerotic plaques, the authors mapped the distribution of macrophages in tissues, and showed that macrophage subsets existed in local metabolic microenvironmental niches. A recent study that Aspect Analytics was involved in examined prostate cancer using spatial lipidomics, and spatial transcriptomics using MALDI imaging and Visium platform, respectively, as well as integrating complmentary sn-RNA seq data and tissue annotations from specialist pathologists8. By combining all these omics techniques and information, the study found unique correlations between lipids and gene expression profiles linked to distinct cell populations and histopathological disease states.
Challenges in spatial multi-omics analyses
It would be wrong of me to say that conducting spatial multi-omics studies is as simple as pushing buttons on a few different machines. Spatial biology data is complex, and generating so much data using different methods, samples, time points, etc. increases the complexity exponentially. As pointed out in this review by Mutuku et al.,9 analyzing these spatial omics datasets requires considerable computing power with statistical tools needed for analysis spread across different programs. Add in that vendors of spatial transcriptomic technology are unlikely to also be vendors for lipidomics and you’ve got a problem for integrating datasets. In addition, since some analyses cannot be conducted using the same tissue (e.g. platforms may require specialized slides or protocols contain steps that digest the tissue), a common co-ordinate system will be needed to conduct differential analysis. So what to do? The studies cited before either developed their own packages for data analysis or customised existing versions, however if you don’t want to or don’t have the ability to build your own software, there is a third option.
Aspect Analytics is actively developing data analysis tools for multimodal spatialomic workflows, such as those that enable the accurate image registration at high spatial resolution. As our software is cloud-based with a web interface, data analysis workflows within the platform are scalable to user needs and can be divided between multiple users. For those who are not yet sure what their data analysis demands are, Aspect Analytics is also available for prototyping and collaborative projects.
Summary
This blog covered some reasons why, how and challenges in spatial multi-omics analysis. Feel free to get in touch with us if you have a project where spatial multi-omics are used and want to collaborate.
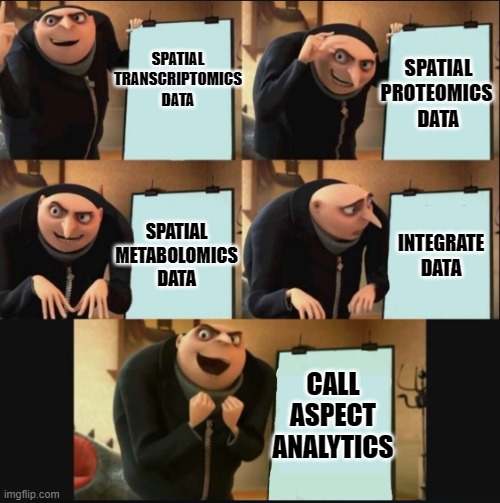
References:
- Crick, F., Central Dogma of Molecular Biology, Nature, 1970. https://doi.org/10.1038/227561a0
- Lempp, et al. Systematic identification of metabolites controlling gene expression in E. coli. Nat Commun, 2019. https://doi.org/10.1038/s41467-019-12474-1
- Carthew RW, Gene Regulation and Cellular Metabolism: An Essential Partnership, Trends Genetics, 2021 https://doi.org/10.1016/j.tig.2020.09.018
- Liu et al, High-Spatial-Resolution Multi-Omics Sequencing via Deterministic Barcoding in Tissue, Cell, 2020. https://doi.org/10.1016/j.cell.2020.10.026
- Stoeckius, Simultaneous epitope and transcriptome measurement in single cells, Nat Methods, 2017. https://doi.org/10.1038/nmeth.4380
- Peterson, Multiplexed quantification of proteins and transcripts in single cells, Nat Biotechnol, 2017 https://doi.org/10.1038/nbt.3973
- Goossens et al, Integrating multiplex immunofluorescent and mass spectrometry imaging to map myeloid heterogeneity in its metabolic and cellular context, Cell Metab, 2022, https://doi.org/10.1016/j.cmet.2022.06.012
- Zhang et al. Integration of Multiple Spatial Omics Modalities Reveals Unique Insights into Molecular Heterogeneity of Prostate Cancer. bioRxiv 2023.08.28.555056; https://doi.org/10.1101/2023.08.28.55505
- Mutuku, Unravelling Prostate Cancer Heterogeneity Using Spatial Approaches to Lipidomics and Transcriptomics, Cancers, 2022, https://doi.org/10.3390/cancers1407170