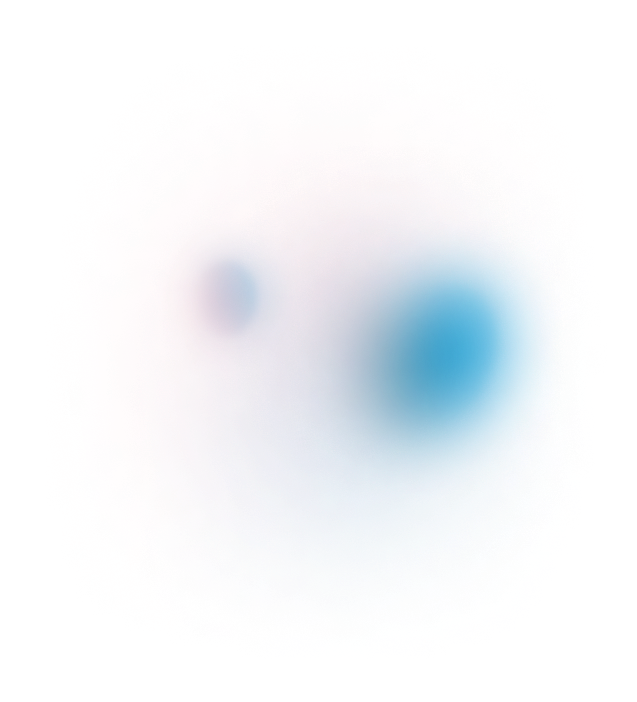
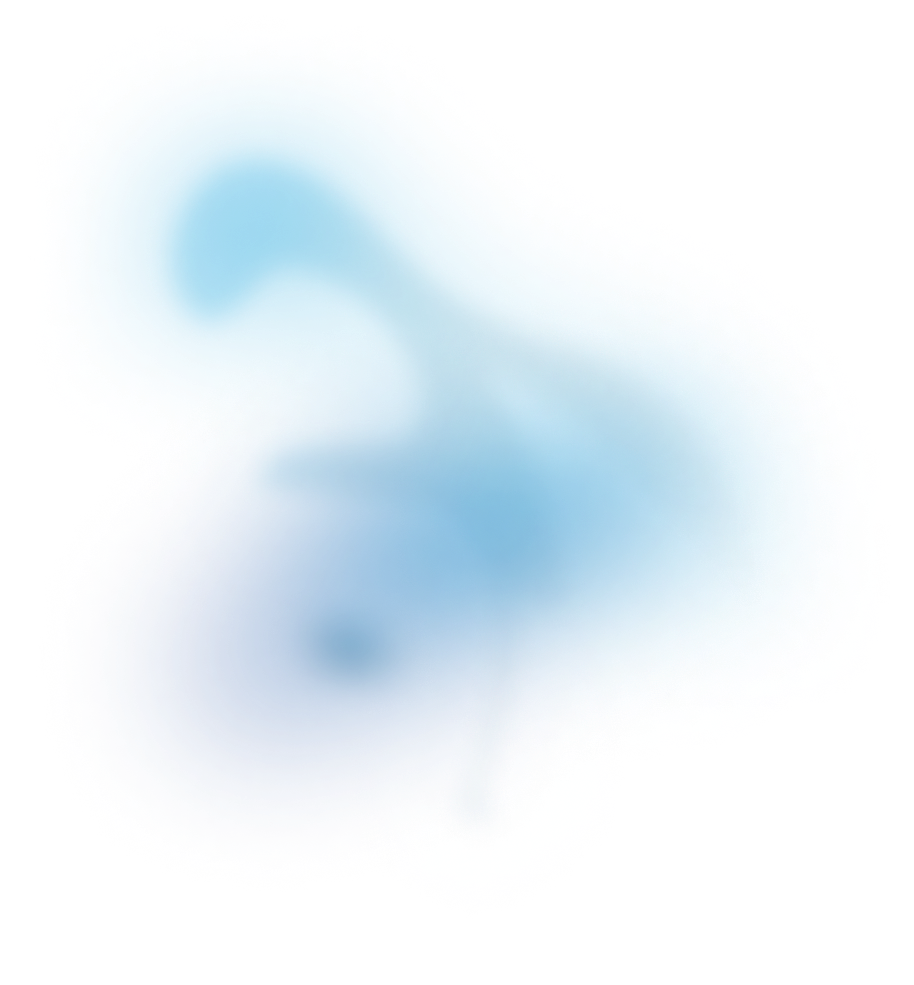
Introduction to spatial multi-omics data analysis
The use of multi-omics technologies is increasingly being adopted to investigate spatial biology questions in health and disease, biomarker discovery and drug development. We provide an introduction to spatial multi-omics data analysis, and the specific opportunities and challenges that can be encountered. This post focuses on the different kinds of readouts from omics technologies and how integration can aid biological research.
Access publication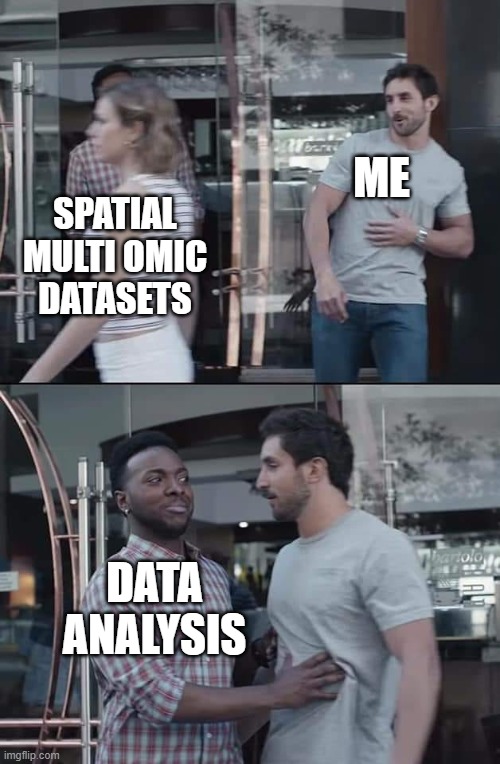
Introduction
As explained previously, multi-omics combines analyses from at least two omics fields (genomics, transcriptomics, proteomics, metabolomics, etc.). Depending on the combination of omics used, these studies can be used to understand the relationship between genotype, phenotype and biological processes in health and disease. Of course, it’s easy to say that omics fields should be combined, but each omics field developed independently of each other, uses different methods and produces different readouts and downstream analyses. So how to get started on spatial multi-omics data analysis?
How spatial multi-omics data integration can expand the understanding of biology
It’s been said before, but it bears repeating – by combining different spatialomics analyses and then integrating them together to better understand associations between genotype and phenotype, we can find novel biological associations and mechanisms underlying disease development and progression. This can lead to a greater understanding of patient response to treatment and the better development of future medications.
While Crick’s Central Dogma is elegant and easy to conceptualize, it is known that one omic level alone is not a guarantee of expression. This is particularly true at the RNA transcript to protein level, with one 2019 study finding that hundreds of proteins could not be detected despite high expression of corresponding mRNA1. This is not trivial – an example of a marker where the transcript and protein levels didn’t correspond is CD82, and I don't think I need to say how important it is to know expression of that protein. For even more examples, here’s an entire twitter thread
Of course, there are also the omics – metabolomics, lipidomics, phosphoproteomics etc, which can’t be predicted by Central Dogma, but can be used to see how environmental changes affect metabolic behaviour, such as this investigation of salinity-induced stress on lipid expression3. But integrating omics data isn’t restricted solely to two different omics techniques – it can also provide an additional layer of molecular information to existing anatomical resources. Verbeeck and colleagues previously linked spatial lipidomics data to the Allen Mouse Brain atlas4, while the Allen Institute’s own Spatial Transcriptomics team themselves proudly announced recently that they had imaged over 600 brain sections.
Spatialomics data formats
But let’s take a step back because before we can talk about integrating data from different spatial biology modalities, it is necessary to know the different formats that might be encountered in this space.
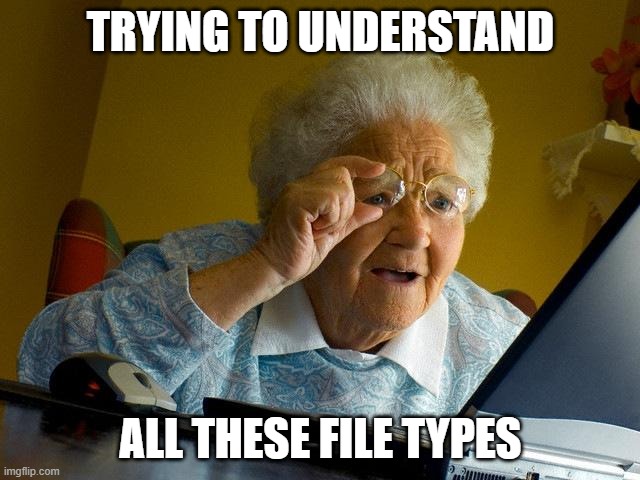
For spatial genomics and spatial transcriptomics, data formats primarily depend on whether the technique is sequencing-based or imaging-based.
- For sequencing-based technology where transcripts are spatially captured but sequenced ex vivo, the output comes from the sequencer used, e.g. Illumina BCL file, which is then converted into the FASTQ format to be ready by downstream analysis tools being used5. However, you will often need a microscopy image for spatial context. Microscopy files will depend on the scanner used e.g. proprietary formats such as MRXS or SVS from Mirax and Leica, respectively, or open formats (e.g. TIFF or OME-TIFF).
- The file formats for Imaging based spatial transcriptomics platforms such as Vizgen’s MERSCOPE and 10X’s Xenium usually consists of JSON and/or CSV files with data on the panels used and genes detected.
Likewise for spatial proteomics techniques, the data format will depend on whether the technique used is microscopy or mass spectrometry-based.
- Outputs from microscopy-based technologies are image files which, again, varies according to platform and vendor, such as the open OME-TIFF format from the Lunaphore COMET, or proprietary QPTIFF used by Akoya instruments.
- Mass spectrometry output files are usually in vendor-proprietary formats, e.g. Thermo RAW files, but this might also depend on the instrument and experiment type. For example, the Bruker timsTOF fleX BAF format for LC-MS/MS experiments, TSF files for MALDI, and TDF when using ion mobility6. There are also vendor-neutral formats such as mzML, developed by HUPO-PSI (Human Proteome Organization-Proteomics Standards Initiative)7 and imzML for mass spec imaging8 with converters available.
Spatial metabolomics, lipidomics and glycomics
- These are primarily investigated using mass spectrometry or mass spectrometry imaging, so vendor formats, e.g. the before mentioned Bruker TSF and kbd files from the Shimadzu iMScope, or the open imzML largely abound here.
As you can tell by the large variety of formats, each field, and in some cases the tech within the field, developed separately, which can be a problem with integration. While open data formats are becoming increasingly available, and is great news for bioinformaticians and other researchers with programming capabilities, where does it leave people who don’t have the capabilities, nor the time or desire to learn to do so? Likewise, what about the people who might be experts in genomics, but might not be great at identifying differences in tissue morphology or knowing different structures?
I can tell you that the first step of integrating spatial biology datasets and interpretation is aligning them, to be covered in the next blog entry.
Summary
This blog discussed some challenges that can be encountered in spatial multi-omics data analysis. Feel free to get in touch with us if you want to integrate and analyze different spatial multi-omics datasets.
References
- Wang D, Eraslan B, Wieland T, A deep proteome and transcriptome abundance atlas of 29 healthy human tissues. Mol Syst Biol. 2019 https://doi.org/10.15252/msb.20188503
- Nicolet BP, Wolkers MC. The relationship of mRNA with protein expression in CD8+ T cells associates with gene class and gene characteristics. PLoS One. 2022 https://doi.org/10.1371/journal.pone.0276294
- Gupta S, Rupasinghe T, Callahan DL,. Spatio-Temporal Metabolite and Elemental Profiling of Salt Stressed Barley Seeds During Initial Stages of Germination by MALDI-MSI and µ-XRF Spectrometry. Front Plant Sci. 2019. https://doi.org/10.3389/fpls.2019.01139
- Verbeeck N, Yang J, De Moor B, et al. Automated anatomical interpretation of ion distributions in tissue: linking imaging mass spectrometry to curated atlases. Anal Chem. 2014. https://doi.org/10.1021/ac502838t
- Liu B, Li, Y, Zhang, L. Analysis and Visualization of Spatial Transcriptomics Data. Front Genet, 2022. https://doi.org/10.3389/fgene.2021.785290
- Luu GT, Freitas MA, Lizama-Chamu I, et al., TIMSCONVERT: a workflow to convert trapped ion mobility data to open data formats, Bioinformatics, 2022. https://doi.org/10.1093/bioinformatics/btac419
- Deutsch E, mzML: A single, unifying data format for mass spectrometer output. Proteomics, 2008. https://doi.org/10.1002/pmic.200890049
- Schramm T, Hester Z, Klinkert I, et al. imzML--a common data format for the flexible exchange and processing of mass spectrometry imaging data". J Proteomics, 2012. https://doi.org/10.1016/j.jprot.2012.07.026