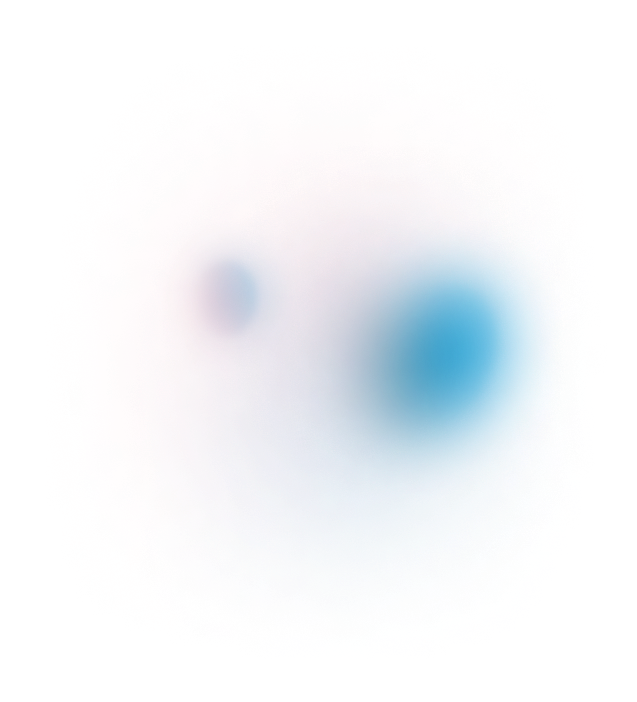
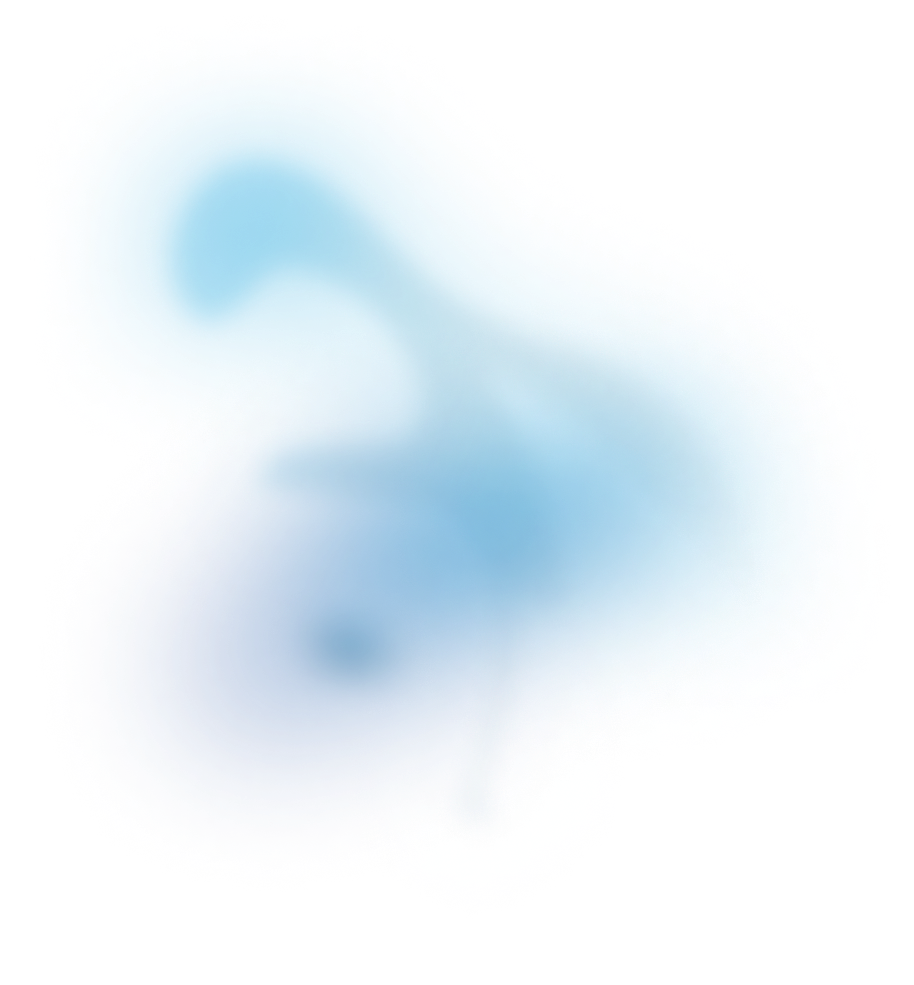
Incorporating morphology via deep learning improves classification performance of MALDI imaging for skin lesions
This presentation addressed how combined analysis of mass spectrometry imaging data and digitized H&E histological images produced more accurate classification skin samples versus approaches that used either the histological images or MSI data on their own.
Access publication
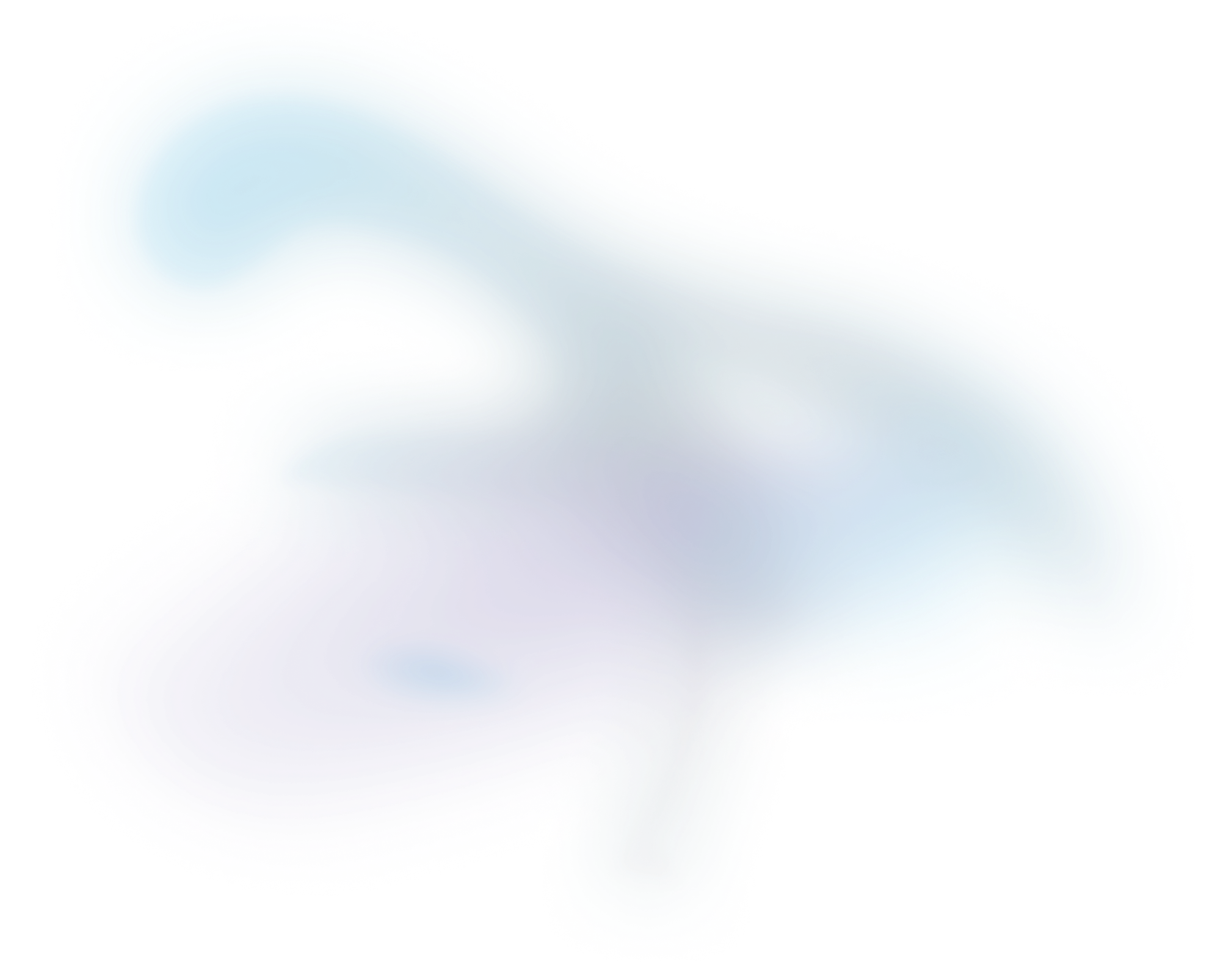
This presentation addressed how combined analysis of mass spectrometry imaging data and digitized H&E histological images produced more accurate classification skin samples versus approaches that used either the histological images or MSI data on their own.

Dermatopathologists annotated digital H&E images of melanocytic lesions as either melanoma, a type of skin cancer, or nevus. These annotations were used to guide MALDI imaging experiments. Data analysis strategies, including using a pre-trained deep neural network, were applied to classify benign nevi samples from melanoma using individually either the H&E images and the MSI data, and then from the fused H&E and MSI data. The results showed that the multimodal pipeline improved both classification performance and stability. This study provides an example where specialized bioinformatics produced better results than a more typical approach.
VIEW SLIDES
If you want to learn more about this work, feel free to contact us.
Publication details
Wanqiu Zhang1,2, Nathan Heath Patterson3,4, Nico Verbeeck2, Jessica Moore3, Alice Ly2, Richard M. Caprioli3,4, Bart De Moor1, Jeremy L. Norris3,4, Marc Claesen2. Incorporating morphology via deep learning improves classification performance of MALDI imaging for skin lesions , 2022
AFFILIATIONS:
- KU LEUVEN, ESAT-STADIUS, LEUVEN, BELGIUM
- ASPECT ANALYTICS NV, GENK, BELGIUM
- FRONTIER DIAGNOSTICS, LLC, NASHVILLE, TENNESSEE
- MASS SPECTROMETRY RESEARCH CENTER, DEPARTMENT OF BIOCHEMISTRY, VANDERBILT UNIVERSITY, NASHVILLE, TENNESSEE